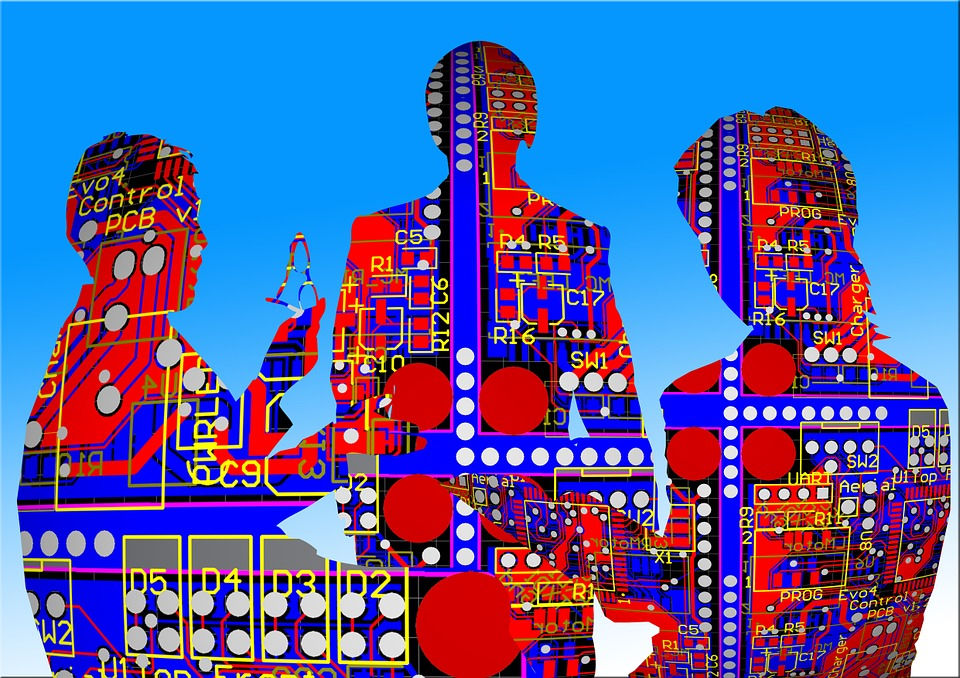
Ever since Geoffrey Hinton, who is considered the father of deep learning, said in a conference of radiologists that the field will soon be taken over by AI(Artificial Intelligence), a huge debate has erupted among radiologists and AI experts, whether this is a possibility in the foreseeable future or not. If we look closely, the answer is clearly; No. Radiologists will not be replaced by AI systems. However, the nature of the job of radiologists will change. The reason why there is confusion in the first place is that radiology(majorly) is a branch of medicine. And medicine is largely misunderstood beyond the practitioners of this field. Furthermore, there are very few people in the world who have a good knowledge of both AI and medicine and also statistical inference associated with it. In classical texts, medical diagnosis is always described as a protocol where a set of procedures are followed. Firstly, a patient presents with a history. The doctor has few diagnoses in mind, based on it. The doctor then carries out physical examination, ruling out some of the diagnoses from the list of differential diagnoses he first had in mind. He further orders diagnostic tests which may include radiological tests to further rule out or confirm the definitive diagnosis. This procedure doesn’t highlight the cognitive or more explicitly, the inferential foundations of medical diagnosis. To generally define it, diagnosis is actually classification on a finite set of classes. These classes are disease states. Unlike Machine learning, clinicians have classically not only used statistical relations between features(eg. signs and symptoms) but also knowledge of the physics of normal and abnormal states (physiology and pathology, respectively) to arrive at a diagnosis or more aptly to classify a patient as to belonging to a given diseased state(s). Suppose a 60 year old male patient comes to the doctor’s office complaining of fatigue, shortness of breath when he walks a few steps and cough. A doctor will not look at what probability the above symptoms appear together in a patient of heart failure or pulmonary hypertension. The doctor will right away try to incorporate these symptoms in the pathophysiology(physics of the diseased state) of few diseased states and then actively seek evidence that will enable him to finally classify the patient into one or more of the diseased states. He/she will subconsciously, follow the same process of incorporation, every few steps of the diagnostic protocol. Radiological evidence also adds up to this process and the major role of the radiologist is to help the clinician in subjectively viewing the evidence in context of the disease model in question. We will see how this subjectivity is important for classification here, but first let us take a look at how an AI system will try to classify a similar problem. AI is a blanket term for number of algorithms but if we generalize it, it boils down to a procedure of weighting variables and transforming them from the dataset which will facilitate the correct outcome. In deep learning for example, the transformation of variables in a variable space(feature space) depends on the architecture of the network and weights are adjusted according to the penalty(cost) if the deep neural network makes a mistake in identifying the correct outcome(derivative of the cost function). So if enough data and its outcomes are fed into this AI system, the system will eventually ‘learn’ to transform variables and weighting them in a way that they can ‘compute’ the correct outcome if an unknown data point is fed into it with the same variables. For example, if images of a chest X-Ray from a patients with pneumonia are fed into a Neural Net where each individual pixel of an image serve as a variable (usually Convolutional neural nets are used for such a task, where variables are ‘patches’ of the image rather than individual pixels) and the weight on that variable and how it is mathematically transformed finally compute the outcome that it is a case of pneumonia or not, this type of general model(details excluding for brevity) can be used to perform any sort of classification, provided that a pattern exists and enough data is fed into the system. A point that needs to be highlighted here is that neural nets, which are most applicable of the AI in this context, are analytical ‘black boxes’. Which means that mathematically we cannot know how data is being transformed inside its layers. Or simply put how data is being ‘used’. For example, we cannot know that on a given image of an X-ray of a pneumonia patient, neural net is confounding on ratios of chest wall to heart size, because these are different in children and children are more prone to pneumonia. This will make the result biased, yet this will not lower accuracy. However, if data changes where now we have more adults in the set and an outbreak of atypical pneumonia has occurred in adults, it might abruptly start giving false results. The type of model based thinking(subjectivity) a clinician uses, reduces the amount of data needed to arrive at the definitive diagnosis. This is because once the clinician has arrived at a diagnosis he/she can simply look for sign and symptoms and lab findings that are bare minimum to diagnose the disease(diagnostic criteria) that are published by accredited medical institutions by research. On the other hand an AI system will need data for every inference it makes. In the absence of a model, it may need to revise it’s data set to update the weights on any new set of population the data is being applied to. A radiologist, in contrast, uses a pathophysiological model to reason, to rule in and rule out many diseased states. Also for testing a machine learning algorithm, data requirement is calculated based on the hoeffding bound. It is a loose bound that ensures that beyond a certain sample size, error will remain very low. Because it is loose, it needs a considerably large dataset to satisfy. And in most instances it is impossible to get a good, random, representative data to perform classification on and be confident that it is not biased. Because of these issues and many such which might arise if we try to make AI systems as stand alone applications, we will always need a ‘supervisor’ who will understand, not only the disease process but also the one who could actually read and use evidence to come up with conclusions and incorporating those conclusion in expanding medical protocols and diagnostic criteria. We are going to need a radiologist who could establish the line of proof and clarify things in larger context. This means that the role of a radiologist will change. The radiologist of tomorrow will have to make sure that AI classifies, what it claims on classifying and understand it in and out so that he/she may use it properly. And also on maintaining subjectivity, so that a clinician can also use these inferences generated through AI algorithms.
Comments